Case Studies: Pharmaceutical

Case Studies
Pharmaceutical
Case Study: Optimizing Drug Quality Assurance: AI-Powered Peak Detection and Integration in HPLC Reduces QA Timelines in the Pharmaceutical Industry
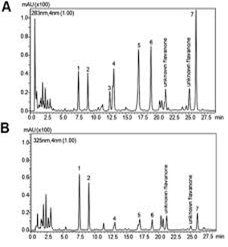
Executive Summary​
This case study explores the implementation of an AI-based vision model for automating peak detection and integration in high-performance liquid chromatography (HPLC) used in drug quality assurance (QA). The AI solution reduced manual intervention, significantly cutting down QA timelines, improving the accuracy of results, and enabling faster drug approval processes within the pharmaceutical industry.
Background​
In the pharmaceutical industry, HPLC is a fundamental technique for analysing drug purity. However, the manual process of peak detection and integration was slow and prone to human error. As part of a digital transformation initiative, our pharmaceutical customer integrated an AI-driven model to automate and optimize these tasks, resulting in substantial improvements in efficiency.Â
Problem Statement​
Manual peak detection and integration in HPLC chromatograms were time-consuming and dependent on human expertise. Errors in peak integration could lead to inaccurate results, delays in product release, and regulatory compliance challenges.Â
Solution:​
An AI-based vision model was implemented to automate the peak detection and integration process. The AI system learned from a large set of chromatogram data and was able to identify peaks with high accuracy and efficiency. Integrated into existing HPLC systems, it reduced reliance on manual intervention.
Implementation
The AI solution was deployed over a 3-month period, integrating seamlessly with the company’s HPLC software. Training sessions were conducted for QA staff to ensure smooth adoption. Data scientists collaborated with the company’s QA team to refine the model for optimal performance and regulatory compliance.
Results and Outcomes
The AI system reduced peak detection time from 4 hours to just 30 minutes per sample, a 90% reduction in processing time. Accuracy improved by 15%, and QA timelines were significantly shortened, leading to faster drug release cycles and increased competitiveness in the market.
Challenges and Lessons Learned
Initial challenges included training the AI model with diverse chromatogram data and ensuring compliance with regulatory standards. The project highlighted the importance of continuous validation and system optimization.
Conclusion
The AI-powered peak detection and integration solution revolutionized the company’s QA processes, reducing timelines and improving product quality. Looking ahead, the company plans to expand AI applications to other areas of drug testing, further improving efficiency across its operations.
Future Recommendations
Future steps include expanding the AI solution to other QA processes and exploring predictive capabilities for potential chromatogram issues. Scaling the technology globally across multiple facilities is also a key priority.